Process Modelling
Advanced manufacturing processes involve phenomena occurring across multiple scales, from macroscopic to atomistic. We have published extensive modelling works on tool/abrasive/workpiece interactions by means of computational fluid dynamics (CFD), discrete element modelling (DEM), continuum mechanics (CM), quasi-continuum (QC) and molecular dynamics (MD). We have also filed 6 patents relating to abrasive processing methods, including shape adaptive grinding (SAG), bonnet polishing (BP) and fluid jet polishing (FJP). We have demonstrated the ability to consistently reach sub-nanometer surface roughness on a wide variety of materials including optical glasses, single and poly-crystals, hard-to-machine metal alloys and ceramics, while being at the forefront of sustainable manufacture by efficient use of hybrid approaches. For example, our originally developed and patented ultrasonic cavitation assisted FJP process can achieve removal rates 1-order of magnitude greater than conventional FJP, while maintaining the same level of surface roughness.
Process Planning
Recently, we initiated a research program on digital twins for advanced manufacturing processes, through the use of machine learning and data mining. The novelty of our approach involves the hybridization of physical models with machine learning algorithms. Using process data from grinding and polishing accumulated over 15 years, a gradient boosting decision tree (GBDT) was used to process a mixture of raw and analyzed parameters pertaining to the material, tool and machine data. By means of particle swarm optimization (PSO), we then implemented a system for automatic selection of process parameters. In experimental trials, our hybrid model vastly out-performs experienced human operators and machine learning “only” algorithms in terms of productivity.
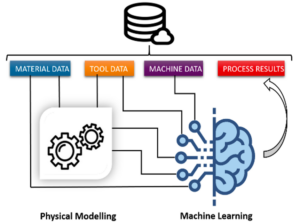
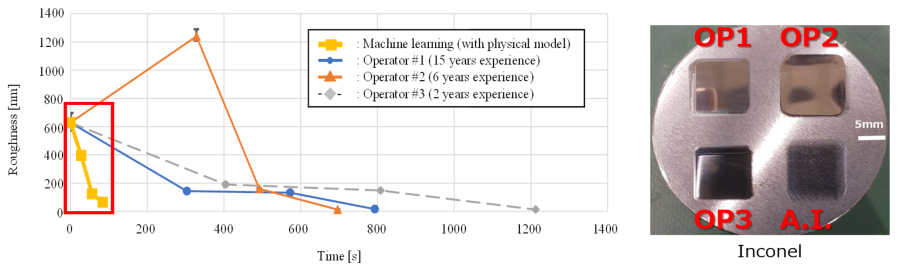